Insurance fraud is a significant challenge that costs the insurance industry $308 billion annually. Fraudulent activities range from individuals exaggerating claims to organized fraud rings orchestrating elaborate schemes. Detecting and preventing insurance fraud is a complex task, requiring advanced tools and techniques.
One such powerful tool is data analytics. Data analytics leverages the power of advanced analytical methods, artificial intelligence, and machine learning algorithms to analyze vast amounts of data and uncover suspicious patterns, anomalies, and connections.
In this blog, we will explore the role of data analytics in detecting insurance fraud and highlight the importance of data quality, integrity, and predictive modeling.
Evolution of Insurance Fraud Detection
To understand how far insurance fraud detection has come, you first need to know where it began. In the past, traditional fraud detection models followed this process:
-
Identification: Fraud detection teams would identify potentially fraudulent claims.
-
Analysis: Special investigation units and claims adjusters would analyze the identified suspicious claims.
-
Recommendation: Upon confirming fraudulent activity, a recommendation would be made to either deny the claim (if detected early) or pursue legal action.
This approach was highly time-consuming and expensive for insurers since claims were frequently settled before conclusive evidence of fraud could be established.
Insurance fraud analytics is accelerating the pace and enhancing the precision of fraud detection. By harnessing the capabilities of artificial intelligence (AI), machine learning, and predictive modeling, insurers can now swiftly identify suspicious behavior and proactively safeguard themselves against fraudulent claims.
These technologies collaborate to continuously learn and automatically identify claims that exhibit similar patterns to previous instances of fraud. Moreover, fraud analytics can employ region-specific AI modeling to detect prevalent fraud schemes and patterns based on the laws and practices specific to that area. This analytical approach offers numerous advantages compared to conventional methods of fraud detection
How Can Data Analytics Help in Detecting Insurance Fraud?
Data analytics is crucial in detecting insurance fraud by leveraging advanced techniques to analyze vast amounts of data and uncover suspicious patterns or anomalies. Here are some ways in which data analytics can aid in detecting insurance fraud:
Pattern Recognition
Data analytics tools can identify patterns and correlations within large datasets, enabling the detection of fraudulent activities. For example, these tools can identify common characteristics and red flags associated with fraudulent claims by analyzing historical claim data. Patterns can include repeated incidents, unusual claim patterns, or suspicious behavior by policyholders, healthcare providers, or other involved parties.
Anomaly Detection
Data analytics can identify outliers or anomalies within a dataset that deviate from normal patterns. These anomalies may indicate potential fraud or irregularities. For example, using statistical models and machine learning algorithms, data analytics can detect unusual claim amounts, unusual claim types, or discrepancies in policyholder information.
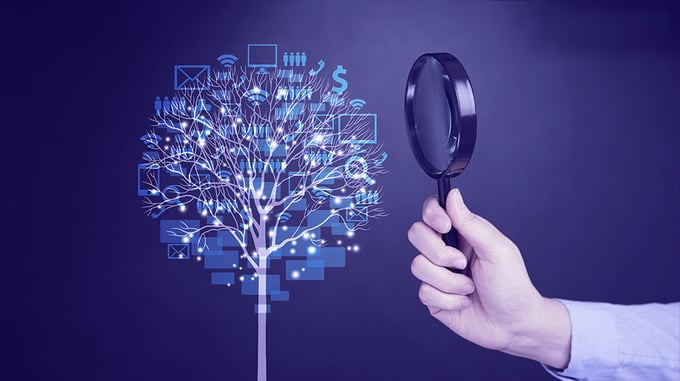
Social Network Analysis
Insurance fraud often involves multiple individuals working together. Data analytics can analyze social networks to identify connections and relationships between individuals involved in suspicious activities. Data analytics can reveal hidden networks or organized fraud rings by analyzing communication patterns, shared addresses, and common healthcare providers.
Predictive Modeling
Data analytics can identify the likelihood of a fraudulent claim by analyzing historical data and applying predictive modeling techniques. Predictive models use machine learning algorithms to assess claim information, policyholder history, and external data sources to generate fraud risk scores. Claims with high-risk scores can be prioritized for further investigation.
Real-Time Monitoring
Data analytics can enable real-time monitoring of claims and transactions, allowing insurers to detect and respond promptly to potential fraud. By implementing automated alert systems, data analytics can flag suspicious activities, such as sudden spikes in claims or policy modifications, enabling fraud detection teams to take immediate action.
.jpg?width=680&height=382&name=Detect-and-Prevent%20(1).jpg)
How Can Data Analytics Help in Detecting Insurance Fraud?
To effectively detect insurance fraud, the quality and integrity of the data are paramount. Data analytics relies on accurate and comprehensive data to identify patterns, anomalies, and fraudulent activities. Therefore, insurers must ensure data quality through rigorous data cleansing, validation, and verification processes.
Data integrity is equally critical, as any manipulation or tampering can undermine the accuracy and reliability of fraud detection efforts. By establishing robust data governance frameworks, insurers can maintain data quality and integrity, enabling more accurate fraud detection outcomes.
The Role of Predictive Modeling in Insurance Fraud Detection
Predictive modeling is a powerful tool in the fight against insurance fraud. By employing machine learning algorithms and statistical techniques, insurers can analyze vast amounts of data to predict the likelihood of fraud. For example, predictive models consider claim information, policyholder history, and external data sources to generate fraud risk scores.
Claims with high-risk scores can be prioritized for further investigation, enabling insurers to focus their resources on potentially fraudulent activities. In addition, predictive modeling empowers insurers to make data-driven decisions, enhance fraud detection accuracy, and minimize false positives, improving operational efficiency and reducing financial losses.
In conclusion, the role of data analytics in insurance fraud detection is undeniably transformative. By harnessing the power of advanced analytical techniques and leveraging the vast amounts of data available, insurers can now detect fraudulent activities more accurately and efficiently. From uncovering patterns and anomalies to assessing fraud risks and implementing real-time monitoring, data analytics empowers insurers to stay one step ahead of fraudsters.
With Pilotbird, the possibilities for fraud detection are expanded even further, allowing insurers to protect their bottom line, maintain the trust of policyholders, and ensure the integrity of the insurance industry. Insurance fraud detection's future lies in data analytics, and Pilotbird is at the forefront of this revolution. Discover comprehensive insights on fraud monitoring by exploring our informative blog.
References
“How AI Data Analytics Helps with Fraud Detection in Insurance Claims?” TestingXperts, 2022
https://www.testingxperts.com/blog/ai-data-analytics-insurance-claims#:~:text=Role%20of%20AI%20data%20analytics%20in%20the%20insurance%20industry,As%20the%20endless&text=AI%20helps%20insurance%20companies%20detect,better%20control%20over%20fraud%20prevention.
"How AI, data analytics could help identify insurance fraud." Insurtech Insights, 2022
https://www.insurtechinsights.com/how-ai-data-analytics-could-help-identify-insurance-fraud/
"8 Benefits of Insurance Fraud Analytics." Duck Creek Technologies, 2022
https://www.duckcreek.com/blog/insurance-fraud-analytics/#:~:text=These%20algorithms%20can%20also%20identify,claims%20or%20potentially%20fraudulent%20claims.
“News & Events.” Conroy Simberg, 2023
https://www.conroysimberg.com/blog/insurance-fraud-costs-the-u-s-308-billion-annually/#:~:text=The%20Coalition%20Against%20Insurance%20Fraud,insurance%20fraud%20has%20been%20updated.
Leave a Comment
Your email address will not be published. Required fields are marked *