In today's complex and dynamic insurance landscape, claims fraud is one constant challenge. The insurance industry has long grappled with fraudulent activities that drain resources, inflate premiums, and undermine trust in the system. As a result, implementing robust fraud detection mechanisms has become imperative for insurance companies worldwide.
This blog delves into the crucial role of claims fraud detection in the insurance industry and explores how cutting-edge technologies and data analytics transform the fight against fraudulent claims.
By effectively identifying and combatting fraud, insurers can protect their bottom line, ensure fair compensation for genuine claimants, and foster a more transparent and reliable insurance ecosystem.
Introduction to Claims Fraud Detection
In the fast-paced world of insurance, where billions of dollars are paid out in claims yearly, the threat of fraudulent activities looms large. Claims fraud can significantly impact insurance companies, leading to increased premiums, financial losses, and erosion of customer trust.
The insurance industry has turned to technology and analytics to mitigate these risks. For example, claims fraud detection has emerged as a crucial tool in identifying and preventing fraudulent claims, ensuring the integrity of the insurance system.
Manual Claims Review
Insurance companies have relied on manual claims review processes for years to detect potential fraud. This method involved skilled claims adjusters carefully scrutinizing each claim to identify suspicious patterns, irregularities, or inconsistencies. While the manual review has merits, it is time-consuming and prone to human error. In an era of technological advancements, insurers turn to innovative approaches for efficient and accurate fraud detection.
Data Analytics and Machine Learning
In 2020, the U.S. insurance sector boasted an impressive value of $1.28 trillion, solidifying its position as one of the world's largest markets. The continuous influx of high premium volumes indicates that the American insurance industry shows no signs of deceleration. With this colossal amount of premiums, an immense volume of data is inevitably involved.
Effectively processing such a vast dataset would become nearly impossible for insurance companies without artificial intelligence technology, particularly machine learning. Failing to leverage these advanced technologies would not only impede efficient data processing but also create fertile ground for the occurrence of insurance fraud.
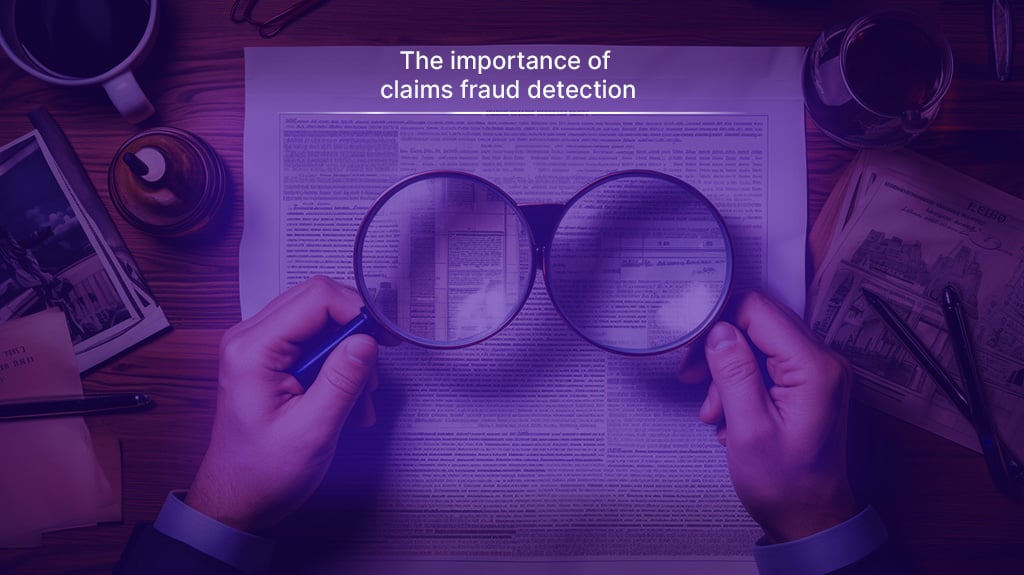
The rise of data analytics and machine learning has revolutionized the claims fraud detection landscape. By leveraging vast amounts of historical claims data and employing advanced algorithms, insurers can quickly identify patterns, anomalies, and potential fraud indicators that may be difficult to detect manually. These technologies analyze multiple variables, such as policyholder information, claim history, and external data sources, to flag suspicious claims and prioritize investigation efforts.
Common Indicators of Fraudulent Claims
As reported by the Coalition Against Insurance Fraud, the annual cost of insurance fraud amounts to a staggering $308.6 billion, causing detrimental impacts on consumers, business owners, and insurance companies alike. Every day, businesses face the looming threat of insurance fraud, encompassing various forms, such as slips and fall and workers' compensation claims.
The risk of fraud knows no bounds and affects enterprises of all sizes. Yet, surprisingly, even small businesses with fewer than 100 employees experience the same median loss from fraud as organizations with 10,000 or more employees.
Fraudulent claims can take various forms, from embellished or fictitious losses to organized criminal activities. While each case is unique, several common indicators can raise red flags for potential fraud:
Property Damage Doesn't Match Injuries
One of the key indicators of a fraudulent claim is when the reported property damage doesn't align with the reported injuries. For example, if a claimant alleges significant bodily injuries resulting from a minor fender bender, it raises suspicion. However, in genuine cases, the severity of injuries is typically proportional to the extent of property damage.
History of Prior Claims
A claimant with a frequent or suspicious claims history can be a red flag for insurance fraud. Insurers often analyze an individual's claims history to identify excessive claims or a pattern of repeatedly filing similar claims. This history may indicate a tendency to exploit insurance policies for personal gain.
Situational Clues
Examining the circumstances surrounding a claim can provide valuable insights. For example, situational clues, such as the absence of witnesses, conflicting statements from involved parties, or unexplained delays in reporting the incident, can raise suspicion. Additionally, extra scrutiny may be warranted if the incident occurred in a location notorious for insurance fraud.
Familiarity with the Claims Process
A claimant with in-depth knowledge of the insurance claims process, including specific terminology, documentation requirements, and timelines, may indicate fraudulent activity. In addition, this level of familiarity suggests that the claimant has prior experience with insurance claims or may have received guidance on maximizing their claim.
Different Versions of What Happened
When multiple parties involved in an incident present conflicting versions of what occurred, it raises concerns about the claim's credibility. Inconsistencies in statements, witness testimonies, or the claimant's own account can indicate an attempt to manipulate the facts to support an inflated or fabricated claim.
Evident Falsification of Injuries
A clear sign of fraudulent activity is when the claimant provides evidence of falsified injuries. This can include forged medical records, exaggerated symptoms, inconsistent medical diagnoses from different healthcare providers, or an unusually long recovery period for minor injuries. In such cases, insurers may employ specialized investigators or medical experts to validate the legitimacy of the claimed injuries.
Developing a Fraud Detection Strategy
In today's increasingly digitized world, businesses face growing challenges in safeguarding against fraud. Therefore, a robust fraud detection strategy is essential to protect the company and its customers from financial losses and reputational damage. Here are a few key considerations when developing such a strategy:
Leverage Predictive Analytics for Early Detection
Early detection of fraud is crucial to minimize potential losses and mitigate risks. One effective approach to detecting fraud is by leveraging predictive analytics. By leveraging sophisticated algorithms and historical data, predictive analytics can detect patterns and anomalies that could potentially signal fraudulent activities.
To implement predictive analytics for fraud detection, organizations can gather and analyze various data types, such as financial transactions, customer behavior, and employee activities.
By applying machine learning techniques, predictive models can be trained to identify suspicious patterns and predict potential fraudulent behavior. In addition, these models can generate alerts or trigger further investigation when a potential fraud event is detected, allowing organizations to take proactive measures in real time.
Analyze Industrywide Data
Fraudsters often target multiple organizations within the same industry. Therefore, analyzing industrywide data can provide valuable insights and help identify fraudulent activities that may go unnoticed within a single organization. In addition, collaborative efforts among industry peers can enhance fraud detection capabilities by sharing anonymized data and patterns of fraudulent behavior.
Industrywide data analysis can be facilitated through data-sharing platforms or industry associations that promote information exchange on fraud trends, techniques, and prevention strategies. By pooling resources and knowledge, organizations can stay one step ahead of fraudsters and develop more effective countermeasures.
Detect Digital Fraud with Image Forensics
As fraudsters become increasingly sophisticated, traditional fraud detection methods may not be sufficient to detect emerging forms of fraud, especially in the digital realm. One powerful technique for combating digital fraud is image forensics. Image forensics involves the analysis of images, screenshots, or visual content to uncover tampering, forgery, or manipulation.
By leveraging image forensics tools and techniques, organizations can verify the authenticity of digital documents, invoices, receipts, or any visual evidence related to transactions. In addition, image forensics algorithms can identify alterations, hidden information, or inconsistencies that indicate fraudulent activities. Integrating image forensics capabilities into fraud detection systems adds a layer of security and helps organizations detect digital fraud more accurately.
Access External Records Quickly
Timeliness is a crucial factor in fraud detection and prevention. Organizations should strive to access external records quickly to expedite investigations and enhance fraud detection accuracy. External records can provide valuable information that supplements internal data, enabling a comprehensive analysis of potential fraud cases.
Collaborating with external data providers, regulatory bodies, or law enforcement agencies can grant organizations access to relevant databases, public records, or blacklists. Integrating external data sources with internal systems allows for real-time checks against known fraudulent entities, suspicious addresses, or flagged individuals. Rapid access to external records improves fraud detection efforts' efficiency and effectiveness.
Embrace Automation in Case Management
Fraud detection involves managing vast information, investigating suspicious cases, and documenting findings. Adopting automation in case management can significantly streamline these processes, improve operational efficiency, and reduce human error.
Automated case management systems can assist in handling alerts generated by fraud detection algorithms, assigning cases to investigators, and tracking the progress of investigations. Workflow automation can enable seamless collaboration between multiple stakeholders in fraud detection, facilitating information sharing, task assignment, and status updates. Additionally, automation can assist in generating reports and analytics to provide valuable insights into fraud trends and patterns.
By embracing automation in case management, organizations can expedite investigations, enhance communication, and focus their resources on high-priority cases, ultimately strengthening their fraud detection capabilities.
Safeguard Your Insurance Future with Pilotbird's Fraud Detection Solutions
Secure your insurance future with Pilotbird's cutting-edge fraud detection solutions. We utilize advanced algorithms and data analytics to identify and prevent fraudulent activities, safeguarding your business and policyholders. Our powerful system quickly detects anomalies, suspicious claims, and patterns, minimizing losses and protecting your bottom line.
With Pilotbird, you can trust that your insurance operations are fortified against fraudulent behavior, ensuring a reliable and secure future. Stay one step ahead of fraudsters with our innovative solutions. Contact us today for a tailored fraud detection strategy that meets your needs.
References
"How machine learning can mitigate the risk of insurance fraud." InsuranceNewsNet, 2022
https://insurancenewsnet.com/innarticle/how-machine-learning-can-mitigate-the-risk-of-insurance-fraud
"Potential signs of insurance fraud." State Auto Insurance, 2022
https://www.stateauto.com/insurance-fraud-signs
"What are Some Common Insurance Fraud Indicators?." Safer America, 2020
https://safer-america.com/what-are-some-common-insurance-fraud-indicators/
"Five data-driven strategies for fighting insurance claims fraud." Verisk, 2020
https://www.verisk.com/insurance/visualize/five-data-driven-strategies-for-fighting-insurance-claims-fraud/
Leave a Comment
Your email address will not be published. Required fields are marked *