The life insurance industry has long struggled with challenging fundamentals. Intense competition erodes value across the board, and only a few industry leaders turn a profit. Compounding the industry's struggles, reports indicate that the global life insurance industry experienced an increase in reported claims to $5.5 billion during the first nine months of 2021 from $3.5 billion for the whole of 2020 due to the COVID-19 pandemic.
In this turbulent environment, enhancing underwriting performance is one proven way to boost competitiveness. But how can insurers achieve this? The use of data analytics capabilities is becoming table stakes in life insurance underwriting. It gives life insurance carriers the opportunity to gain valuable intelligence and insights to streamline processes, optimize resources, and improve profitability. In fact, according to a Willis Towers Watson study, life insurance carriers who leverage predictive analytics, experience a 60% increase in sales and a 67% reduction in expenses.
In this post, we'll review key steps for implementing better insurance data analytics in life insurance underwriting.
How to Implement Better Insurance Data Analytics in Life Insurance Underwriting
More and more insurers are investing in data analytics solutions and understand that these solutions are key to surviving in the rapidly evolving environment. Even so, a recent study found 90% of insurance companies struggle to see a positive case of data analytics solutions. This is mostly due to the poor implementation of insurance data analytics solutions.
Below is an outline of the steps that insurers can use to optimize data analytics in life insurance underwriting:
1. Problem Framing and Identification of Business Goals
Data analytics in life insurance underwriting typically begins with an insurer focusing on solving a given problem, such as reducing fraudulent claims or improving customer recommendations. Identify the most promising insurance products, determine which products and riders should be in the scope of the analysis, and define the relevant hypothesis to be tested.
Your focus should be on clarifying a specific goal using measurable outcomes. Insurers may collaborate with statisticians to establish the metrics for measuring success. It's also crucial to place the goals in a broader context.
2. Data Extraction, Cleaning, and Crunching
Data is usually collected and stored in multiple systems, which require expert knowledge to extract data from. Insurers should be able to identify the data that is relevant to the underwriting process. They should be able to determine what data sets are available and how this data can be used to improve predictions and address other business goals. Once data is extracted, it is transformed and combined into an integral analysis dataset for validation and modeling purposes.
That said, the relevance, suitability, quality, and cleanliness of data must be considered. Understanding how and why data is collected can help carriers identify any problems in advance prior to feeding it into their data analytics model.
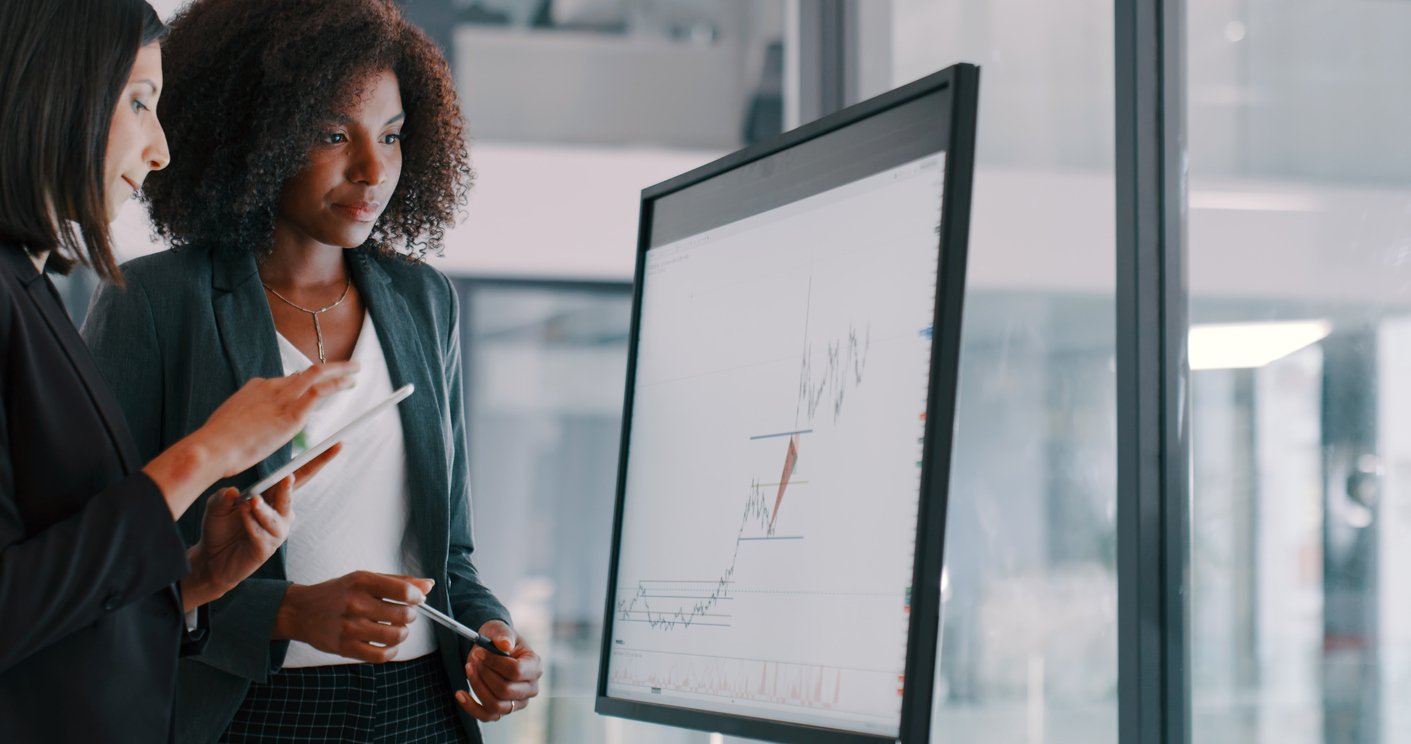
3. Development of the Model
Consider how different data analysis models might yield the most valuable outcome, and then work backward to determine the most ideal way of organizing the raw data into an appropriate set of features for the model. Experiment with different algorithms, features, and processes that strike the right balance between accuracy, performance, and any other aspects like explainability. Life insurers can use a data wrangling tool to transform raw data into more conclusive data sets for calculating a prediction.
Most insurers prefer using predictive and descriptive underwriting and pricing models since they are built using quantitative and qualitative knowledge that considers all the business domains relevant to the analytics use case.
4. Deploy the Model to Support and Automate Business Decisions
The newly developed data analytics model is then put into action to see if it can deliver results. Insurance data analytics insights are only valuable if they make a meaningful difference in the underwriting process. It should, for instance, be able to help insurers determine how risk-averse an insured is. This way, they'll be better placed in determining whether or not someone qualifies to take a life insurance cover and, if so, what premiums they should pay.
5. Monitoring the Model to Compare Its Performance to the Expected Results
Suppose the data science was well executed, the data analytics model should be able to meet the accuracy, performance, and other requirements when it is deployed with live data. Even so, performance can change over time.
In some cases, especially when extracting information from social media, potential policyholders may subvert the accuracy of the data analytics model by deliberately divulging misleading information on their social media accounts. In other cases, the data analytics model helps insurers obtain useful insights that they can use to close the loop in product design in the following ways:
- Extra-premium loadings that don't offer a significant premium increase versus claims should undergo re-evaluation by the actuarial team. The premiums can be either increased or converted into an exclusion.
- Extra-premium loadings that generate more premiums compared to their relative claims can be kept as-is or reduced to improve the competitive edge.
- Exclusions that don't provide sufficient protection against claims can be scrapped in the underwriting process to reduce costs and improve operational speed.
That said, upon the deployment of the model, continuous monitoring is required to ascertain whether it's meeting performance expectations. The work isn't complete here, though, given that there may be a need to redefine the model to ensure it stays in line with the changing life insurance industry market dynamics.
How Insurance Data Analytics Is Used in the Life Insurance Industry
Insurance data analytics is an extension of the techniques that insurers have used for several years to calculate risks and set premiums for their insurance policies. The manual underwriting techniques that insurers relied on in the past years are expensive and time-consuming.
Insurance data analytics tools used in life insurance streamline the underwriting process and improve risk assessment, which maximizes the profitability of the insurers. Besides that, it also improves their customer retention rates. For instance, using these tools together with genetic profiling can help life insurance companies distinguish risks related to unhealthy lifestyles from those associated with genetic factors that are beyond the policyholder's control and adjust the policy accordingly.
If you are looking to improve your underwriting process, look no further than Pilotbird's insurance data analytics solutions. These solutions have features such as scoring for assessment of lifestyle risk, hit rate for confidence level, plus it's designed for efficient use by teams. Contact us for a free demo of our insurance data analytics solutions.
REFERENCES
Dekkers, J. & Choudhury, A. (n.d). Insurance analytics: Applying analytics in the underwriting process. Deloitte. https://www2.deloitte.com/nl/nl/pages/financial-services/articles/applying-insurance-analytics-in-the-underwriting-process.html
Maryville University. (n.d). Predictive Analytics in Insurance: Types, tools, and the future. https://online.maryville.edu/blog/predictive-analytics-in-insurance/
Willis Watson Towers Survey. (2019, July 8). The financial rationale for predictive analytics. https://www.wtwco.com/en-US/Insights/2019/07/the-financial-rationale-for-predictive-analytics
Diemer, S. (n.d). Stay on top of your performance with insurance analytics. https://www.datapine.com/insurance-analytics
BusinessWire. (2020, July 1). Turning data into insights with commercial analytics. https://www.businesswire.com/news/home/20200701005334/en/Turning-Data-Into-Insights-With-Commercial-Analytics-Quantzig%E2%80%99s-Recent-Article-Reveals-Three-Best-Practices-for-Insurers
Cohn, C. & Hussain, Z. (2022, January 13). Life insurers adapt pandemic risk models after claims jump. https://www.reuters.com/business/life-insurers-adapt-pandemic-risk-models-after-claims-jump-2022-01-13/
Leave a Comment
Your email address will not be published. Required fields are marked *